To know how a system performs across a range of AI workloads, you look at its MLPerf benchmark numbers. AI is rapidly evolving, with generative AI workloads becoming increasingly prominent, and MLPerf is evolving with the industry. Its new MLPerf Training v3.0 benchmark suite introduces new tests for recommendation engines and large language model (LLM) training.
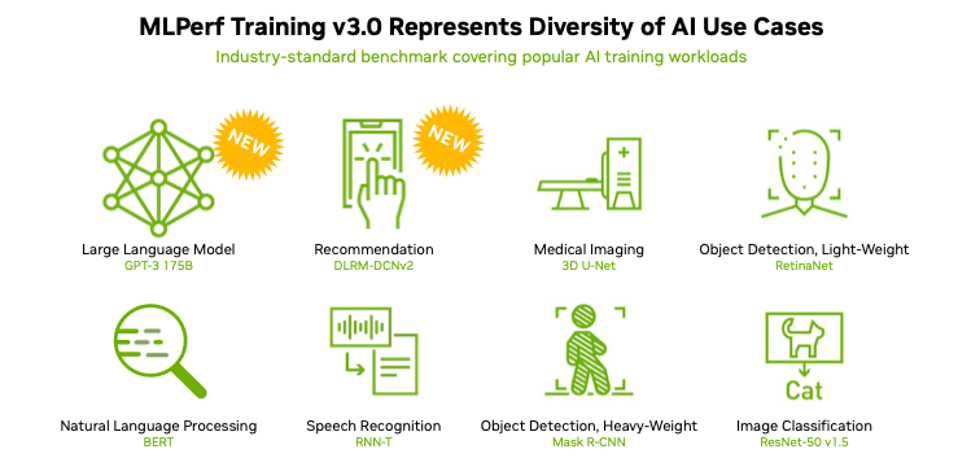
MLCommons, which oversees MLPerf, released the latest MLPerf benchmark results today. The NVIDIA H100 dominated nearly every category and was the only GPU used in the new LLM benchmarks.
The top numbers for LLM and BERT natural language processing (NLP) benchmarks belonged to a system jointly developed by NVIDIA and Inflection AI and hosted by CoreWeave, a cloud services provider specializing in enterprise-scale GPU-accelerated workloads. To say that the numbers are impressive is an understatement.
NVIDIA H100 Dominates Every Benchmark
The MLPerf LLM benchmark is based on OpenAI’s GPT-3 LLM trained with 175 billion parameters (GPT-3 was the latest generation GPT available when the benchmark was created last year). Training LLMs is a computationally expensive task, with Lambda Labs estimating that training GPT-3 with 175 billion parameters requires about 3.14E23 FLOPS of computing. That’s a lot of expensive horsepower.
NVIDIA designed the H100 Tensor Core GPU for exactly these workloads, and it is quickly becoming one of the most popular accelerators for training large language models. This is for a good reason. NVIDIA introduced a new transformer engine in the H100, explicitly designed to accelerate transformer model training and inference (NVIDIA provides an excellent description of the device’s full capabilities in a blog post). Transformers are at the heart of generative AI, so it’s expected that the H100 should perform better than previous generations. NVIDIA says everything is faster on the H100, with its new transformer engine boosting training up to 6x.
Of the 90 systems included in today’s results, 82 used NVIDIA accelerators (of the 8 non-NVIDIA systems tested, all but one were submitted by Intel). Just under half of all results were based on the NVIDIA H100 Tensor Core GPU. The NVIDIA H100 set records on every workload in the MLPerf training and inference benchmarks, while NVIDIA’s A100 and L4 GPUs provided healthy inference results.
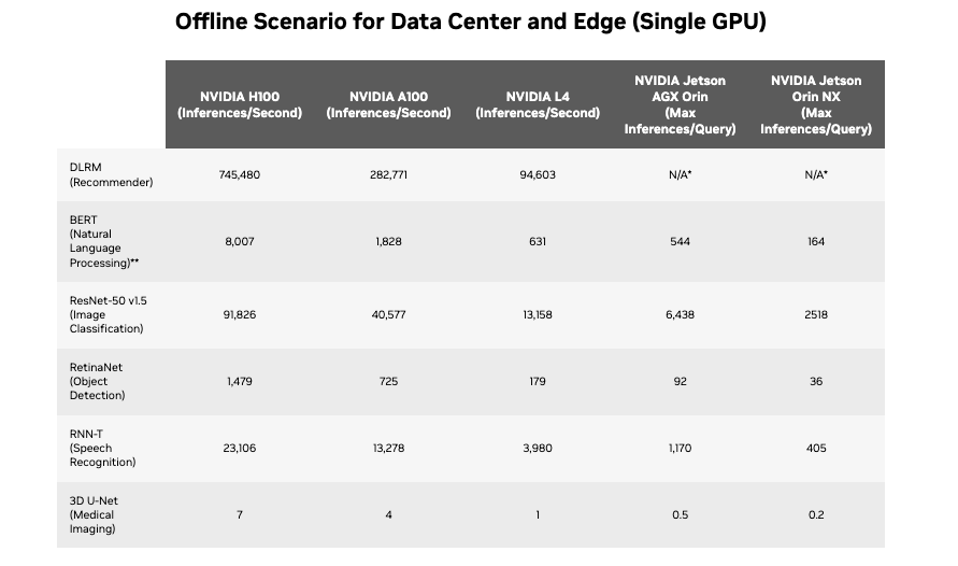
Looking deeper into the metrics, the NVIDIA H100 Tensor Core GPU yielded a per-accelerator LLM training time of 548 hours (about 23 days). The GPU also set per-accelerator records on every benchmark tested.
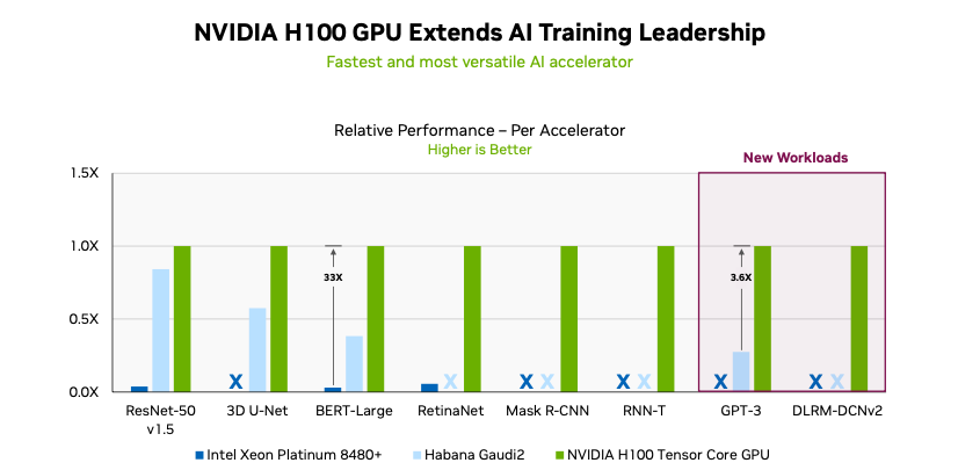
LLM at Scale: NVIDIA + Inflection AI + CoreWeave
Looking at per-accelerator results is interesting, but real-world production workloads are rarely built using single accelerators. There are efficiencies of scale that emerge in a clustered system with multiple GPUs, something NVIDIA designed in from the start with its ongoing focus on GPU-to-GPU communication using its NVLink technology. Understanding real-world performance requires looking at results on a system level.
NVIDIA and Inflection AI co-developed a large-scale GPU cluster based on the NVIDIA H100 Tensor Core GPU, hosted and tested by Coreweave. The system combines 3,584 NVIDIA H100 accelerators with 896 4th generation Intel Xeon Platinum 8462Y+ processors. The results are staggering, setting new records on every workload tested.
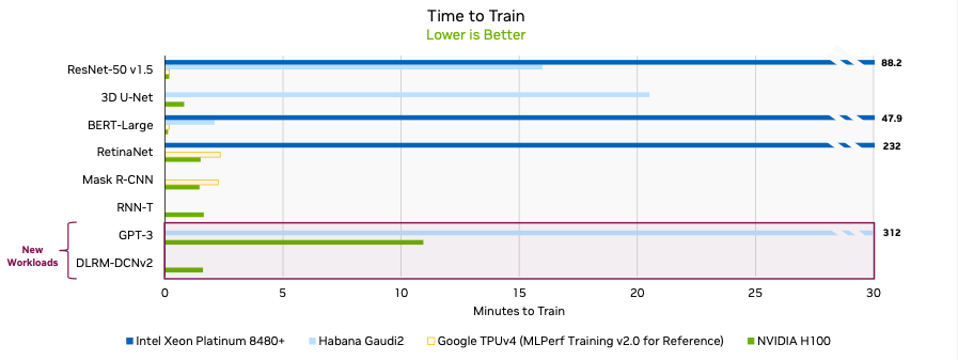
Delving into the LLM benchmarks shows off the full capabilities of NVIDIA’s technology. The 3,854 GPU cluster completed the massive GPT-3-based training benchmark in less than eleven minutes, while a configuration containing half that number of GPUs completed in nearly 24 minutes, demonstrating the non-linear scalability potential of the NVIDIA H100 GPU.
Intel was the only other entity to report LLM benchmark results. Intel’s systems combined 64-96 Intel Xeon Platinum 8380 processors with 256-389 Intel Habana Gaudi2 accelerators. Intel reported LLM training times of 311 minutes for its top-end configuration.
Analysis
Benchmarks provide a point-in-time comparison of systems. That nearly every submitted result was based on an NVIDIA accelerator speaks to the continued dominance of NVIDIA within the AI ecosystem. While this dominance is largely based on its accelerator technology, the stickiness of NVIDIA within the ecosystem is still very much governed by the reliance on its software by the AI community.
NVIDIA doesn’t just provide the low-level CUDA libraries and tools upon which nearly every AI framework is based, the company has moved up the stack to provide full-stack AI tools and solutions. Beyond enabling AI developers, NVIDIA continues to invest in enterprise-level tools for managing workloads and models. NVIDIA’s software investment is unmatched in the industry and will keep NVIDIA in the driver’s seat for the foreseeable future. There will be non-NVIDIA solutions for training, but those will continue to be the exception.
My biggest takeaway from the MLPerf results isn’t the raw performance of NVIDIA’s new H100 Tensor Core Accelerators but rather the power and efficiency of running AI training workloads in the cloud. Building a training cluster of any size is an expensive and complex undertaking. NVIDIA doesn’t release pricing for its H100 accelerator, but it’s estimated to be between $30,000-$40,000/each. CoreWeave will rent one to you for $2.23/hour, delivering training results as well as any on-site installation (in a further plug for CoreWeave, I’ll point out that it’s not yet possible to get time on an H100 from any of the top public cloud providers; no CSP has an H100-based instance generally available today).
AI is changing the way we engage with technology. It’s changing how businesses operate and how we understand the data surrounding us. NVIDIA sits at the center of this revolution, rapidly expanding its presence into nearly every element of the data center. NVIDIA isn’t the gaming graphics company we grew up with. It’s instead quickly becoming a key enabler for our collective future. Keep watching, but they’re just getting started.