Oracle introduced full support for vectors, including vector search, in its just-released Oracle Database 23ai. Known as AI Vector Search, this capability represents a significant advancement in how databases can store, index, and search data semantically.
Vector search is a powerful computing technique that performs searches based on the similarity of data points represented as vectors in a multidimensional space. Unlike traditional search methods that rely on keyword matching or exact data matches, vector search allows for identifying items based on contextual similarity. This approach is beneficial for handling unstructured data, such as text, images, audio, and video, enabling more nuanced and intelligent search capabilities.
Beyond its ability to expand the value of data with contextual search, vector search also lies at the heart of tailoring generative AI and large language models (LLMs) to the enterprise’s specific needs.
This Research Paper, sponsored by Oracle Corporation, delves into the new capability.
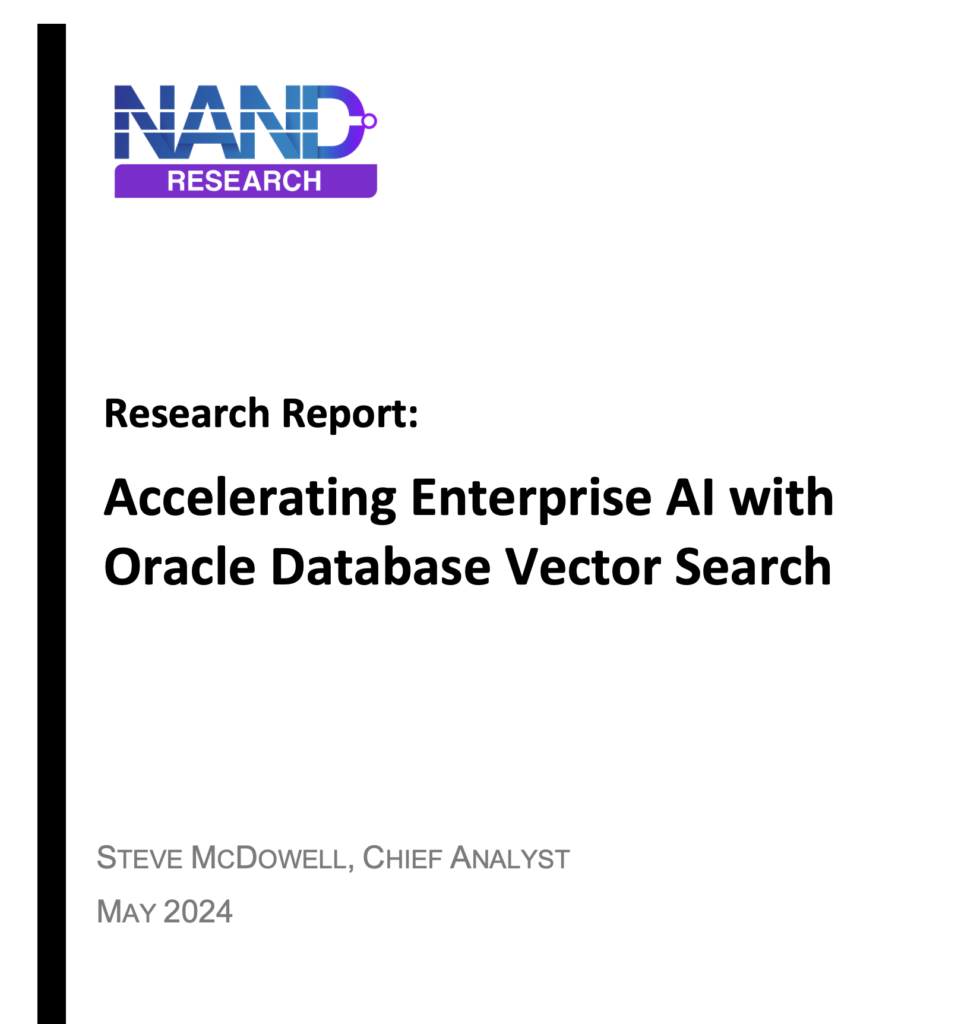